What does the future of data science mean for everything from location and property selection to asset management and disposition? A data veteran weighs in.
In a sense, real estate investing is easy. All you need to do is identify a soon-to-be-hot neighborhood or submarket before anyone else does, acquire the best undervalued properties in the neighborhood, maintain the highest rent rates possible while keeping your properties fully occupied, dispose the moment the market plateaus. And then do it all over again in the next hot neighborhood.
Just follow this recipe and success is guaranteed:
- How do we know that a neighborhood will be hot soon? To the best of my knowledge, time travel hasn’t been invented yet.
- How do we know that the neighborhood will be the hottest of all? If prices in the neighborhood are rising but they are rising even more in other neighborhoods, did we choose the right neighborhood?
- While picking the “best” property in the neighborhood seems doable, how do we know that it is “undervalued?” What is the fair market value of a property? Won’t the property become “overvalued” immediately after the landlord figures out it was “undervalued”?
- The best property in the neighborhood is most probably not for sale, let alone the discount. How would we buy a property that is not being sold?
- How do we win the competition for the best property acquisition, while keeping the price in the “undervalued” range?
- How do we make sure our property is fully occupied? What if it needs some remodeling? What if another better, brighter property is constructed next door?
- How do we make sure we keep the rents high enough but not too high for tenants to start checking out? What is the fair market rent for our property?
- How do we know when the market reaches its plateau?
- How would we find a buyer for our property then, while maintaining the “undervalued” status without actually selling it under its value?
We’re putting aside the fact that the interest rates of today appear to make real estate investing simply not economical. While I can’t control the interest rates (never mind that time travel is not needed to predict that they will go down eventually), in my career, I have come across data-driven solutions to most (not all) problems mentioned above.
Recently, I co-authored two research articles in the AFIRE Summit journal, one of which (with Donal Warde and Prof. Maxime Cohen) dealing with location selection, and the other (with Donal Warde) providing insights into property selection. Those papers partially cover property acquisition questions above, while the latter questions are related to data-driven asset management, in which Cherre, the company where I served as CTO from 2018 to 2022, is a true expert. Property disposition (last two questions) is to an extent similar to property acquisition, as discussed below.
Based on my experience, which is somewhat unique in this industry, let me walk you through the proposed solutions.
LOCATION SELECTION
Yes, it’s hard to predict the future, but we’re in luck: in real estate, things take time to change. If we succeed in recognizing early indicators of the change, we could project them into the future. But what are those early indicators? As I often hear from real estate investors: “If Whole Foods/Starbucks/Shake Shack/pick-your-favorite-food-chain opens in the neighborhood, then it’s time to invest.”
And my response is always the same: “If they opened a Whole Foods in the neighborhood, it’s too late to invest.”
If national corporations recognized the potential of the neighborhood and moved in, the neighborhood had been developing for years already.
Another popular concept of “follow the artists” (see which neighborhood artistic people are moving into) is smart but hardly a strategy, as the phenomenon doesn’t seem to be measurable. What we suggest is rather “follow the money”, or more precisely, see where the wealth is migrating.
In a nutshell, if an average household net worth in the neighborhood is, say, $200,000, and the net worth of families that are moving in is $400,000, then the wealth excess that the new families are bringing would in part be invested/spent/taxed locally, in a way for the neighborhood to eventually enjoy the outcome and become stronger. If ten thousand families live in the neighborhood while only ten wealthier families move in, the influence of their wealth excess would be negligible. If, in contrast, a thousand wealthier families move in, they would probably change the neighborhood quite substantially. How to recognize the wealth inflow trend early enough is the essence of our research.
If we had a nationwide, decades-spanning dataset of family relocations (from address A to address B) which would also incorporate their net worth at the time of the relocation, we would have been able to predict real estate trends quite accurately. Some companies, such as ADP or Intuit, might have a partial view on this data, as they would keep addresses of their customers together with the customers’ financial records. Those companies are not in the real estate space though, and there are many reasons for them not to use their data for predicting real estate trends. (The best source of wealth migration data would undoubtedly be the IRS—and I sincerely hope that they are not supplying their data to real estate investors.)
Given the above, the relocation data should be collected from public sources, which includes real estate transactions stored in county records. When a family sells their property at address A for X dollars and moves to address B, they are likely to bring X dollars with them to the neighborhood where B is located. If the median house price in that neighborhood is 2X, then the family does not seem to bring any wealth excess into the neighborhood. If, however, the median house price in the neighborhood is X/2, this neighborhood is the one to watch for.
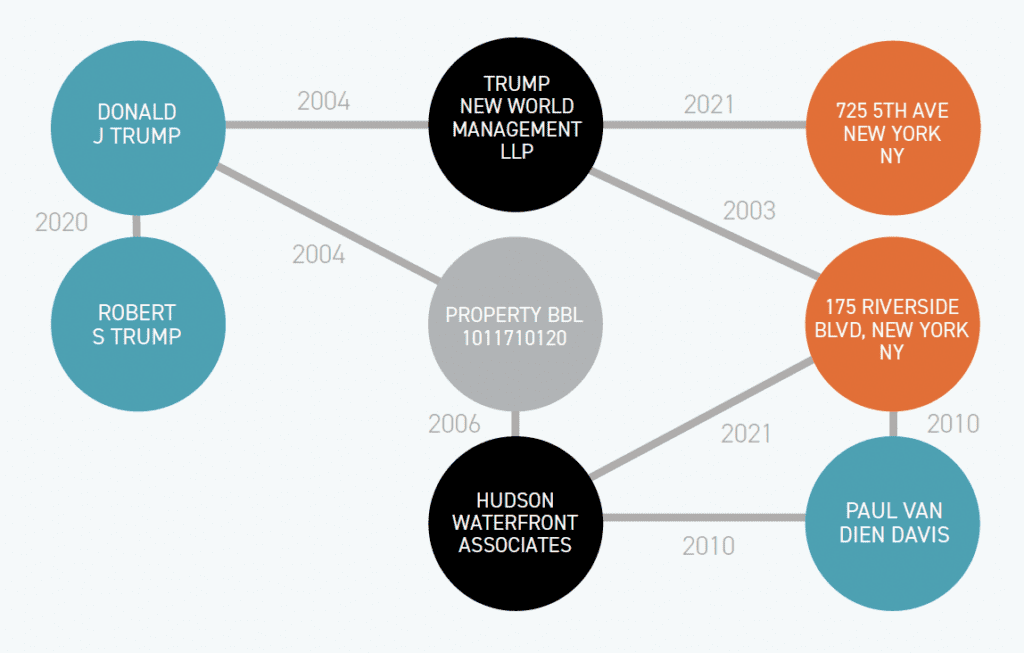
When aggregated over all US neighborhoods and over the past two decades, this data becomes an important index using which neighborhoods can be ranked, compared to each other, and monitored over time. If the wealth migration index starts rising for a certain neighborhood (faster than for other neighborhoods), this should attract investors’ interest even if the neighborhood does not yet show any other signals of a near-future boom.
While being conceptually intuitive, the wealth migration index is notoriously difficult to construct. How do we define a “family” from the data point of view? Did the family actually live in the property that they sold? Did the family actually sell the property at a fair market price? Did the family actually move into the neighborhood? And, above all, is this the same family that sold a property in one neighborhood and moved to another neighborhood? These and many other related questions can be answered with the help of a real estate Knowledge Graph, which is an actionable model of the entire real estate ecosystem. At Cherre, we developed the largest-of-all real estate Knowledge Graph and used it to disambiguate names of real estate owners. This helped us distinguish between the cases of someone moving from address A to address B and the cases of someone moving out of A while their namesake was moving into B.
Needless to say, utilization of the wealth migration index does not eliminate the neighborhood due diligence process that investors would go through. However, it allows a quick scan of thousands of neighborhoods and opens the door to true globalization of real estate investments as the investors will no longer need to stick to the neighborhoods they are overly familiar with. Moreover, the same wealth migration measurement approach can be used at different levels of granularity, starting with US states, down to counties, towns/neighborhoods, and all the way to the level of a block, providing an opportunity of selecting the best location at the national scale.
PROPERTY SELECTION
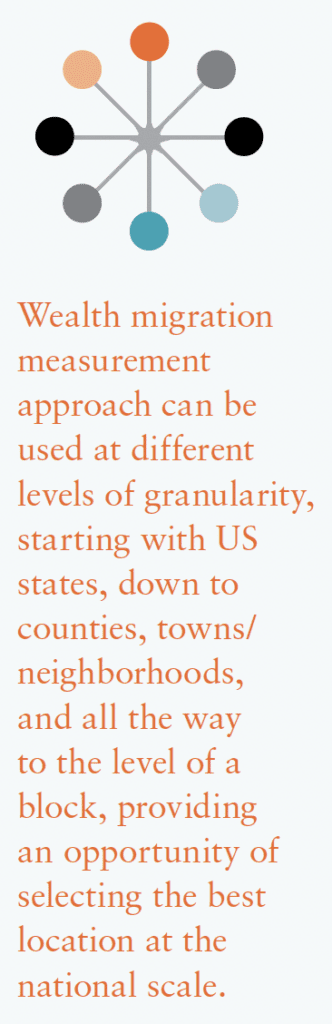
Once the location has been selected, finding the right property is usually not a complex task as every investor has a specific set of criteria. In many cases, there are very few properties in the neighborhood that meet those criteria, and so the acquisition process would start naturally.
Nevertheless, while focusing on property characteristics, investors often overlook a critical factor in the acquisition process: the property owner. Obtaining as much information about the owner as it can be obtained about the property itself would be a valuable tool in the acquisition negotiation. However, the information on the owner is not readily available. Alas, the ownership information is often unavailable: most commercial properties are owned by obscure LLCs. Owner unmasking is a data-driven solution to the problem of detecting true owners behind the LLCs, but unmasking is not enough.
In the ideal world, where a perfect neighborhood can be selected and all qualified properties in the neighborhood can be listed, detailed information on the owner would be pretty much the only piece of information needed to close a deal. Is the owner inclined to sell? If yes, is the owner ready to sell for a discounted price? If we could assess our chances a priori, even before the acquisition process begins, we would be much smarter throughout the process. Moreover, this would open a gate to the holy grail: off-market deals.
Despite the general consensus that off-market deals are most attractive, they are fairly rare. The main reason is the tremendous amount of work that needs to be put into an off-market deal to close. First, find out who the owner is. Find out how to contact them. And if the owner is not interested in selling, now what? Years, if not decades, may be invested in maintaining a working relationship with a potential seller before the dream deal can be finally executed. And what if, in a few years, this deal won’t be a dream deal anymore? Time is money. That’s why investors typically opt for on-market deals and rely on their negotiation skills to craft the best terms within the existing circumstances, all this while being left in the dark about the true intentions of their negotiation counterpart.
Fortunately, data science offers solutions. First, it’s not hard to find out whether the property owner is an individual or a corporation: in many cases, it’s enough to see if the LLC’s mailing address is a private residence or a suite in an office building. Individual owners and corporate owners tend to behave differently in the way they craft their deals. As we established in our recent research work mentioned above, individuals tend to buy cheaper properties but they also tend to obtain lower returns when they sell. This indicates that deals should be negotiated differently for individual sellers compared to corporate ones.
Second, many individual owners of commercial real estate are public figures; a lot of information on them can be retrieved from the internet. Corporations, however, are more difficult to crack, still the list of their executives is often publicly available, which points back to the claim above: many real estate corporate executives are public figures.
Companies such as Usearch that operate in the space of web structurization (extracting unstructured information from the Web and organizing it into databases) may provide valuable insights about property owners, which would be sufficient for understanding their intentions. And this can be done on scale, thousands of owners at a time. All a buyer is left to do is to choose an owner that seems to be ready to talk and use the provided insights wisely in the negotiation process.
There is no such thing as the fair market value of commercial real estate: it’s up to us—the seller and buyer—to agree on the price, and it’s up to us to excel in negotiations.
Data science can help us prepare for the negotiation process, but it is not going to take the human factor out of the equation.
ASSET MANAGEMENT
After the deal is closed and funds are deployed, it’s time for the tedious task of asset management. A large part of the asset management process is focused on monitoring of different key performance indicators, at different levels of granularity: monitoring of neighborhood trends, rent prices, occupancy rates, migration patterns, local employment characteristics, transportation adjustments, municipal legislation, and many other aspects of social development which could directly or indirectly affect the financial health of real estate investments. The starting point of effective monitoring is data aggregation and normalization.
However, monitoring everything everywhere does not seem feasible nor necessary; say, how would a change in gas prices at a local gas station affect our multifamily? That is why asset managers tend to narrow their monitoring efforts down to a few comparable properties (comps). The definition of a comp is usually intuitive to real estate professionals, but the data tells a different story: human intuition may be misleading.
Data-driven comp models select substantially different comps. I am not suggesting to trust the algorithm more than we trust our intuition, but I believe that our vision of the market is quite limited and may be significantly enhanced if we take the algorithm’s results into account. When I showed the results of a data-driven comp model to domain experts, their response was “these comps I know, those are not comps at all, but these are comps that I overlooked.”
IN THIS ISSUE
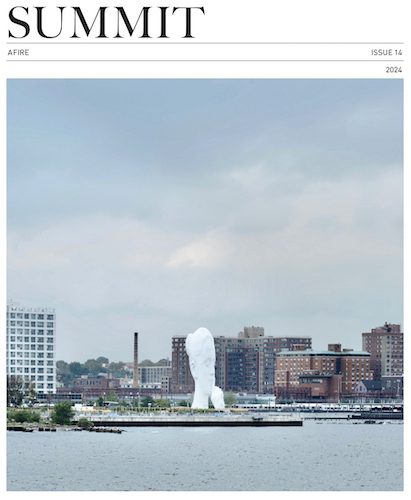
NOTE FROM THE EDITOR: WELCOME TO #14
Benjamin van Loon | AFIRE
INSURING FOR ELSEWHERE: CLIMATE-RESPONSIVE REAL ESTATE INVESTMENT
Benjamin van Loon | AFIRE
INSURING FOR ELSEWHERE: STRIPPING THE CASHFLOW FROM THE DEAL
Paul Fiorilla | Yardi
MARKET OUTLOOK: MODEST GROWTH AND RETREATING INFLATION IN 2024
Martha Peyton, CRE, PhD | LGIM America
UNDERPERFORMANCE PARADOX: NEW RESEARCH QUESTIONS THE VALUE OF PRIVATE REAL ESTATE FUNDS
William Maher, Taylor Mammen, Ben Maslan | RCLCO Fund Advisors
NAVIGATING THE CURVE: RESILIENCE, ADAPTATION, AND PREPARING FOR 2024
Jack Robinson, PhD | Bridge Investment Group
LIQUIDITY FREEZE: POTENTIAL SOLUTIONS FOR COMMERCIAL REAL ESTATE
Christopher Muoio | Madison International Realty
SUPPLY WAVE: REASON FOR OPTIMISM IN THE MULTIFAMILY SECTOR
Sabrina Unger, Britteni Lupe | American Realty Advisors
MANAGE WHAT YOU MEASURE: UNDERSTANDING EXPENSE INFLATION IN APARTMENTS
Gleb Nechayev | Berkshire Residential + Webster Hughes, PhD | ThirtyCapital
PARSING OFFICE DISTRESS: PLANNING FOR THE NEXT GENERATION OF OFFICE SPACE
Dags Chen, Lincoln Janes, CFA | Barings Real Estat
MODEL STATES: USING ECONOMIC STATE MODELS TO ASSESS THE US OFFICE OUTLOOK
Armel Traore Dit Nignan | Principal Real Estate
OUTWARD SHIFT: WILL THE LOGISTICS SECTOR CONTINUE TO OUTPERFORM?
Kerrie Shaw | AXA IM Alts
HARNESSING THE WIND: TECHNOLOGICAL CHANGE AND THE PROMISE AND PERIL OF AI FOR REAL ESTATE
Nikodem Szumilo | University College London + Chris Urwin | Real Global Advantage
MIND YOUR DATA: REAL ESTATE INVESTING, FROM THE POINT OF VIEW OF A DATA NERD
Ron Bekkerman, PhD
OPERATING EXPENSES RISING THE OTHER MAJOR COMPONENT OF NOI GETS MORE FOCUS
Stewart Rubin, Dakota Firenze | New York Life Real Estate Investors
IN MEMORIAM: JANICE STANTON
+ LATEST ISSUE
+ ALL ARTICLES
+ PAST ISSUES
+ LEADERSHIP
+ POLICIES
+ GUIDELINES
+ MEDIA KIT (PDF)
+ CONTACT
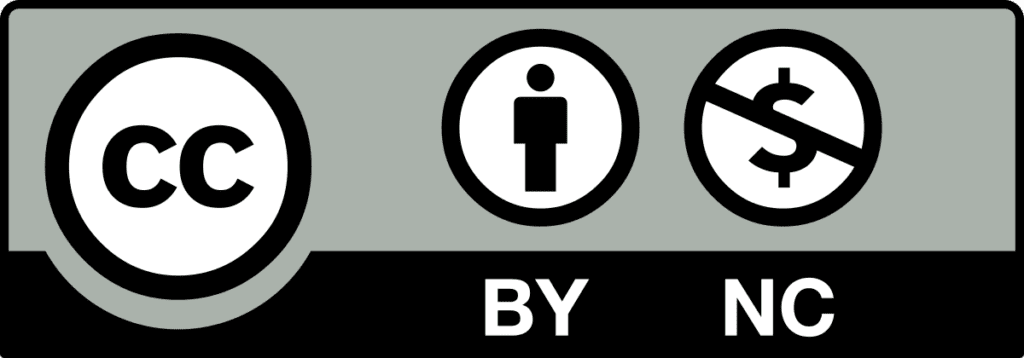
The big advantage of a data-driven comp model is in its ability to bring many more comps than those identified manually. In many cases, an asset manager monitors very few comps, and the conclusions that they make may be statistically invalid. For example, if three comps are being monitored and rent prices are rising in all of them, the asset manager may assume an uptrend. However, if thirty comps were monitored, the asset manager would see that rents were rising for only ten of them, and the three original comps ended up among those ten just by pure chance. Moreover, because scale is simply not an issue for data science, a system can monitor three hundred or even three thousand comps, and aggregate the results proportionally to their distance/similarity to the asset at hand. This would count both global and local trends as one.
PROPERTY DISPOSITION
Typically, assets are sold when the fund ends. There is not much variability in the process, and this sounds like a miss. But what’s right for private equity funds is not necessarily the correct model for family offices and other investment vehicles. And even in private equity cases, timing is not that rigid after all.
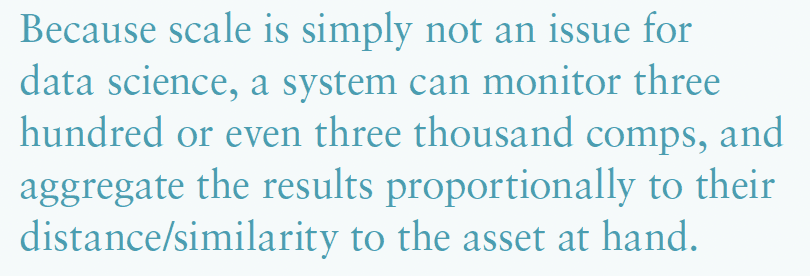
So, when should we sell? First, when the existing assets don’t appear to appreciate any longer, and second, when a better opportunity is around the corner. How do we line these two factors up with the fund lifecycle? We monitor!
As asset managers, we can project the appreciation trend of our assets into the near future, and we can use the wealth migration index described above as a tool for assessing the development trend of our neighborhood.
As the neighborhood gentrifies, it will eventually reach its peak, in the most natural way. Illustrating this on an edge case, assume that everyone in the neighborhood is extremely rich, which means that there are simply too few people in the world who would be richer than the neighborhood’s residents and willing to move in. With time, whoever moves in will on average be poorer than the current residents, and some of the rich residents will move out, so the wealth migration index will start sliding down, even for the best neighborhoods. As the index slides, no signs of deterioration would yet be visible, and may never be. However, prices will eventually plateau at or below the national trend, which might be too late for selling the property. Detecting the upcoming plateau early enough would be crucial for timing the property disposition.
Identifying the next opportunity is yet another key factor at the property disposition stage of the investment cycle. Is there anything more interesting in the market right now, something that would outshine our existing investments? The wealth migration index would help us figure this out, as we discussed earlier. A bright side of the wealth migration index is that it is timely: at every point of time, it lets us select the top locations, which makes it easy to align with the maturity of existing investments. Even if adversarial microeconomic factors make the investment timing suboptimal, we could still focus on best locations for the current conditions. Obviously enough, I could not touch on every aspect of the real estate ecosystem in one article, and there are many other aspects of the real estate investment business in which data science can be instrumental (debt structuring being just one example). But for some real estate processes data science would still be quite useless: people would always be better than machines at tasks such as client relations and negotiations. Nevertheless, it’s time to jump on the Data Science bandwagon, before it gets overcrowded!
—
ABOUT THE AUTHORS
Ron Bekkerman, PhD, is a Strategic Advisor for Cherre.
—